Introduction
Ethical AI refers to the practice of designing, developing, and deploying artificial intelligence with good intention to benefit people and the planet while minimizing harm. It emphasizes the creation of artificial intelligence (AI) systems that are transparent, fair, responsible, and trustworthy. As AI becomes more integrated into our daily lives, the importance of ethics in AI is paramount to address issues like bias, privacy, equality, and accountability. Ethical AI requires collaboration among policymakers, technologists, and stakeholders to establish guidelines and frameworks that govern the ethical use of AI. By prioritizing ethical considerations, we can ensure that AI technologies are aligned with human values and societal well-being.
Points to Note:
- Ethical AI practices are essential to address the ethical implications of AI deployment.
- Evaluating and improving the performance and fairness of language models and AI systems is crucial.
- Bias and fairness in language models pose significant challenges that need to be addressed.
- Explainability plays a vital role in ensuring accountability and transparency in AI decision-making processes.
- Addressing bias at the source, through ethical data usage, is crucial for building unbiased AI systems.
Table of Contents
History of Ethical AI
The history of Ethical AI is a narrative that traces back to the mid-20th century, starting with early philosophical debates about the moral implications of intelligent machines. As AI technology advanced, the 1970s saw the development of foundational theories in computer ethics, addressing the societal impacts of computing technologies.
By the 1990s, ethical considerations had begun to be formally integrated into academic programs related to AI and technology. The importance of ethical oversight became more recognized in the industry around 2016, leading to the establishment of ethics boards and panels by major tech companies.
Year | Milestone | Description |
---|---|---|
1940s-1950s | Early Discussions on AI Ethics | Philosophers and scientists begin debating the moral implications of intelligent machines. |
1970s | Foundational Theories | Theoretical frameworks for computer ethics developed, considering the societal impact of computing. |
1990s | AI Ethics in Academia | Ethical considerations become a topic of study within AI and technology-related academic programs. |
2016 | AI Ethics Boards and Panels | Major tech companies establish ethics boards to oversee the ethical deployment of AI. |
2018 | AI for Good Movement | The AI for Good movement gains momentum, emphasizing AI’s potential to address global challenges. |
2019 | Regulations and Guidelines | Governments and organizations start to implement ethical guidelines and regulations for AI. |
2021 onwards | Continuous Ethical AI Advancements | Ongoing research and discourse on how to ensure AI develops in an ethical and controlled manner. |
The Unveiling Dilemma: Bias and Fairness in Language Models
One of the paramount concerns in the realm of AI ethics is the presence of bias in language models. These models, often trained on large datasets reflective of societal biases, can inadvertently perpetuate and amplify existing prejudices. Evaluating and addressing bias requires employing robust evaluation metrics, such as disparate impact and equalized odds, to gain insights into the fairness of model predictions.
The Role of Explainability in AI Accountability
As AI systems become more complex, ensuring accountability and transparency in decision-making processes is crucial. Explainability, or the ability to understand and interpret the decisions made by AI systems, is a pivotal aspect of accountability. Researchers are actively working on developing explainability techniques, including model-agnostic methods and interpretable model architectures, to enhance the transparency of language models and AI systems.
Enhancing Accountability Through Explainability
In the realm of AI ethics, explainability plays a vital role in holding AI systems accountable for their actions. Imagine a scenario where an AI-driven algorithm denies a loan application. In such cases, it is essential to explain the factors and criteria used to arrive at the decision, ensuring fairness and transparency.
Explainability is a multifaceted concept, encompassing various techniques that shed light on AI system decision-making processes. From post-hoc analysis to the design of inherently transparent models, researchers are actively working towards providing explanations for AI system outputs.
Model-Agnostic Explainability Methods
Model-agnostic techniques aim to provide explanations for AI systems regardless of their underlying architecture. These methods focus on interpreting how these AI models arrive at specific decisions. Techniques like LIME (Local Interpretable Model-Agnostic Explanations) and SHAP (SHapley Additive exPlanations) are commonly used to generate post-hoc explanations for black-box models.
Interpretable Model Architectures
Interpretable model architectures are designed to incorporate transparency from the ground up. These models are specifically built to provide understandable and explainable predictions. Examples include decision trees, rule-based models, and linear models. By prioritizing interpretability during model development, the process of understanding AI system decisions becomes more accessible and transparent.
Model Explainability Techniques | Key Characteristics |
---|---|
Post-hoc Analysis (LIME and SHAP) | – Provide explanations for black-box models – Focus on interpreting model decisions |
Interpretable Model Architectures | – Designed to be transparent from inception – Built-in interpretability for understandable predictions |
The Ethical Implications of Training Data: Addressing Bias at the Source
The roots of bias in language models often lie in the training data used to train these systems. Biased training data can result in models that exhibit discriminatory behavior. To address bias at the source, it is crucial to adopt ethical data collection practices, employ diverse and representative datasets, and continuously monitor and audit training data for potential biases.
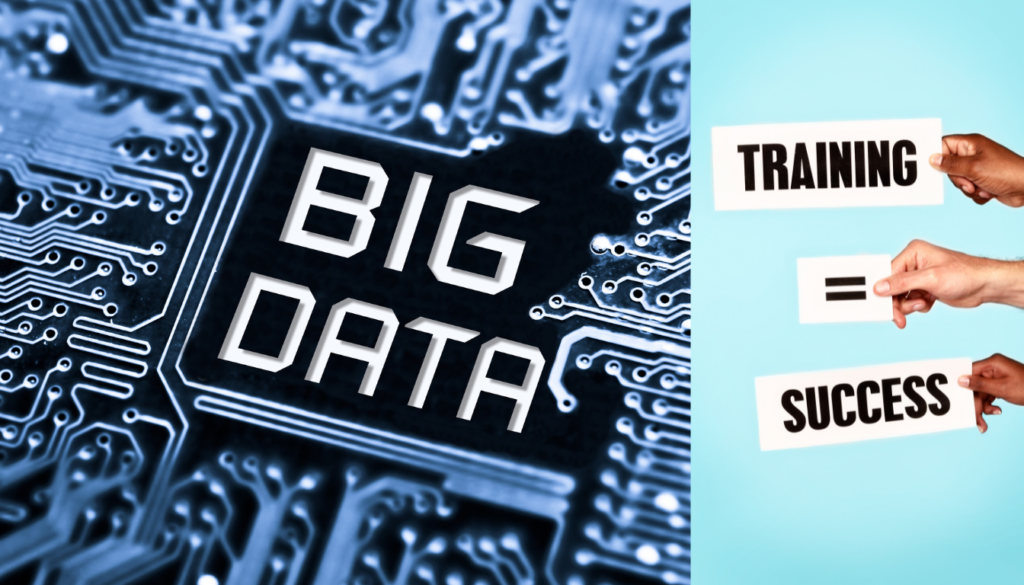
Why Ethical Data Usage Matters
Ethical data usage is a fundamental aspect of responsible AI and machine learning. By ensuring that the training data is unbiased and representative, we can build models that avoid perpetuating existing prejudices or discriminatory behaviors. Ethical data usage encompasses various practices, such as:
- Implementing rigorous data collection methodologies that avoid biases and uphold privacy standards.
- Ensuring diversity and inclusivity in the datasets to mitigate underrepresentation and the amplification of minority biases.
- Regularly monitoring and auditing the training data to identify and rectify any biases that may emerge.
- Establishing transparent guidelines and protocols for data collection, usage, and storage.
Addressing Bias: Ethical Machine Learning
Ethical machine learning plays a crucial role in addressing bias at the source. It involves the implementation of techniques and strategies to identify and mitigate biases in the training data and ensure fair model outcomes. Some key practices in ethical machine learning include:
- Building diverse and representative datasets by considering different demographic groups and perspectives.
- Using pre-processing techniques to detect and mitigate biases in the training data, such as removing sensitive attributes or applying data augmentation techniques.
- Adopting fairness metrics and algorithms to evaluate and minimize the impact of biases in the model’s predictions.
- Applying interpretability methods to understand how the model makes decisions and identify potential sources of bias.
Monitoring and Auditing Training Data: Key Considerations
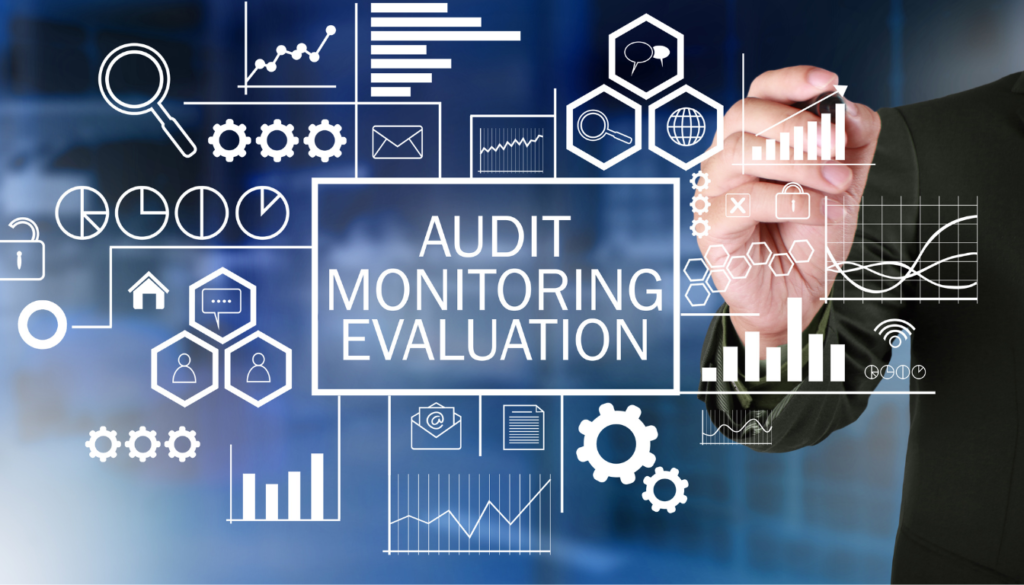
Ongoing monitoring and auditing of training data are essential for maintaining ethical practices and avoiding biases. It is important to establish a comprehensive framework for data auditing that involves the following steps:
- Regularly reviewing and evaluating the quality and representativeness of the training data.
- Conducting bias assessments to identify potential biases emerging from the training data.
- Implementing robust mechanisms for user feedback and engagement to gather insights into potential biases and refine the training data accordingly.
- Developing clear documentation of the data collection and auditing process to ensure transparency and accountability.
By prioritizing ethical data usage and implementing robust practices for monitoring and auditing training data, we can mitigate bias at the source and build fair, transparent, and trustworthy AI systems.
Benefits of Ethical Data Usage | Challenges in Addressing Bias | Key Considerations for Data Monitoring and Auditing |
---|---|---|
– Build fair and unbiased AI systems | – Inherent biases in training data | – Regularly review and evaluate training data quality |
– Avoid perpetuating existing prejudices | – Ensuring diversity and representation in datasets | – Conduct bias assessments |
– Enhance transparency and trustworthiness | – Implementing fairness metrics and algorithms | – Gather user feedback and engagement |
– Uphold ethical principles and guidelines | – Interpreting model decisions to identify bias sources | – Maintain clear documentation of the auditing process |
Real-world Challenges: Current Issues in AI Ethics
Real-world instances of biased AI systems underscore the critical importance of ethical considerations in AI development. These instances serve as stark reminders that the deployment of AI technologies can have far-reaching consequences that need to be carefully addressed.
Biased Algorithms Shaping Hiring Decisions
One notable challenge is the presence of biased algorithms influencing hiring decisions. Research has highlighted instances where AI-driven systems perpetuate gender or racial biases, leading to discriminatory outcomes in the selection process. This raises concerns about equal opportunities, fairness, and diversity in the workforce.
Racial Biases in Facial Recognition Systems
Another pressing issue is the racial biases exhibited by facial recognition systems. Studies have shown that these systems tend to have higher error rates for individuals with darker skin tones, jeopardizing the accuracy, fairness, and trustworthiness of such technologies. The impact of these biases can be far-reaching, affecting areas such as surveillance, law enforcement, and identity verification.
The Importance of Transparency and Accountability
These real-world challenges underscore the crucial need for transparency and accountability in AI development. Stakeholders, including policymakers, researchers, and developers, must work together to ensure that AI systems are built and deployed in an ethically responsible manner. This requires clear guidelines, frameworks, and oversight to prevent and address biases, discrimination, and unfair outcomes.
To navigate the complex landscape of AI ethics, it is essential to promote awareness, education, and dialogue among all stakeholders. By addressing the real-world challenges and proactively seeking solutions, we can pave the way for the responsible and ethical development of AI technologies.
Towards Fair and Responsible AI: Solutions and Recommendations
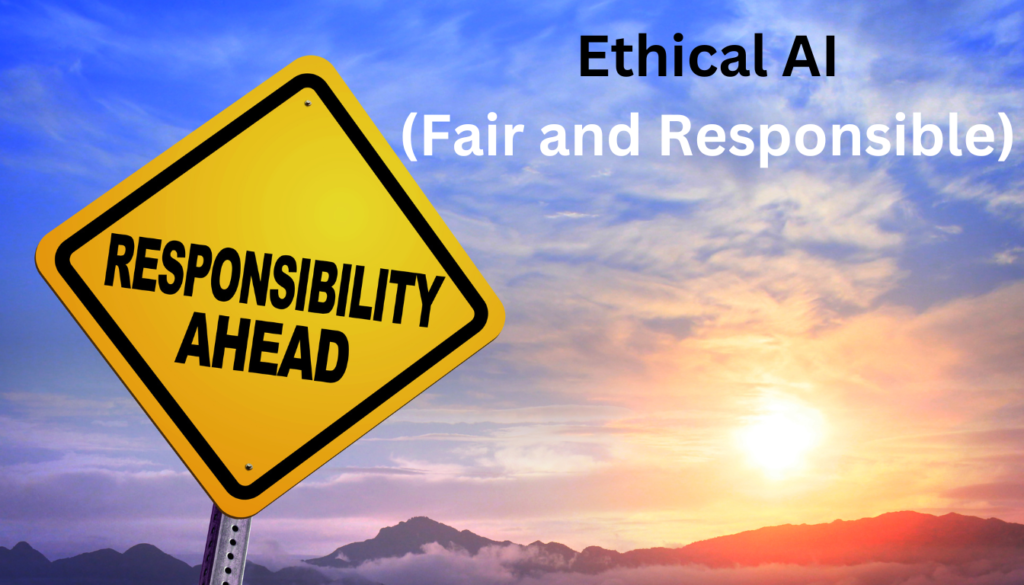
To ensure fair and responsible AI, diversity in development teams is crucial for mitigating bias. By bringing together individuals from different backgrounds and perspectives, ethical AI can be created with a comprehensive and unbiased approach.
Establishing and adhering to ethical guidelines and frameworks is another essential step in promoting fairness, transparency, and accountability in AI systems. These frameworks provide a set of principles and standards that guide the development, deployment, and use of AI technologies.
Ongoing monitoring and auditing of AI systems are necessary to identify and address any biases or ethical concerns that may arise during the AI lifecycle. This includes regular evaluation of the data used for training and testing, as well as continuous monitoring of the system’s performance and impact.
In addition to internal monitoring, public engagement is crucial for building trust and ensuring that AI systems align with societal values and expectations. By involving stakeholders, such as users, communities, and organizations, in the decision-making process, AI developers can gain valuable insights and address any potential ethical concerns.
Finally, conducting algorithmic impact assessments can help evaluate the potential risks and ethical implications of AI systems. These assessments involve analyzing the impact of AI technologies on different stakeholders, considering factors such as fairness, privacy, and safety.
Best Practices for Building Trustworthy AI
Practice | Description |
---|---|
Diversity in Development Teams | Include individuals with diverse backgrounds and perspectives in AI development teams to mitigate bias and promote fairness. |
Adhering to Ethical Guidelines | Establish and follow ethical frameworks and guidelines that prioritize fairness, transparency, and accountability in AI systems. |
Monitoring and Auditing | Regularly monitor and audit AI systems to identify and address any biases or ethical concerns that may arise. |
Public Engagement | Involve stakeholders in the decision-making process to ensure that AI systems align with societal values and expectations. |
Explainability | Promote transparency and accountability by enabling users to understand how AI systems make decisions. |
Algorithmic Impact Assessments | Conduct assessments to evaluate the potential risks and ethical implications of AI systems on different stakeholders. |
Best Practices for Ethical AI Design
When it comes to designing AI systems with ethics at the forefront, there are several best practices that can help guide the approach. By incorporating these practices into ethical AI development process, you can address biases, promote fairness, prioritize data privacy, and ensure human oversight in AI systems.
Diversify Input Data and Training
To minimize bias and broaden the perspectives represented in your AI models, it is crucial to ensure a diverse range of input data. Incorporating data from various sources and demographics can help mitigate unintended biases in the training process.
Ensure Transparency and Accountability
Building trust in AI systems requires transparency in their decision-making processes. Make efforts to provide clear explanations of how the ethical AI system arrives at its predictions or recommendations, making it easier for users and stakeholders to understand and assess the system’s behavior.
Implement Ethical Guidelines and Frameworks
Establishing and adhering to ethical AI guidelines and frameworks can serve as a compass for ethical decision-making. These guidelines can help you navigate complex ethical dilemmas, ensure responsible AI practices, and align your AI development with societal values.
Conduct Ethical Review Boards
Regularly convening ethical review boards can provide valuable insights and perspectives from diverse stakeholders. These boards can help identify potential ethical challenges or biases in AI systems and provide recommendations for addressing them.
By following these best practices, you can create ethical AI systems that not only deliver accurate and reliable results but also incorporate ethical considerations at every stage of development. Ethical AI design empowers users and builds trust in the technology, ultimately leading to more responsible and trustworthy AI systems.
Conclusion and Final Thoughts
In today’s era of rapid technological advancement, the responsible use of artificial intelligence (AI) is paramount. By embracing ethical principles and guidelines, we can harness the power of AI to drive innovation, efficiency, and positive change while safeguarding individual and societal interests.
Addressing bias is a critical aspect of ethical AI. By evaluating and minimizing bias, we can ensure fairness and promote equal opportunity in AI systems. Transparency is another key factor, allowing us to understand and interpret AI decisions, engendering trust and accountability.
Adhering to ethical frameworks and guidelines provides a foundation for responsible AI practices. By continuously monitoring and auditing AI systems, engaging the public, and conducting algorithmic impact assessments, we can prioritize fairness, transparency, and responsible AI deployment.
Upholding ethics in AI is not only essential for mitigating potential harms but also for maintaining trust and stability in the industry. By embracing ethical AI practices and frameworks, we can shape the future of AI to benefit humanity, fostering innovation and progress while working towards a more equitable and inclusive society.
FAQ On Ethical AI
What is Ethical AI?
Ethical AI refers to the practice of developing and deploying artificial intelligence technologies in a manner that is moral, responsible, and aligned with human values. It emphasizes transparency, fairness, accountability, and minimizing harm to individuals and society.
Why is Ethical AI important?
As AI systems become more prevalent in daily life, their decisions can have significant impacts on privacy, employment, safety, and societal norms. Ethical AI ensures that these technologies are used to benefit society, mitigate risks of bias, discrimination, and ensure the rights and values of individuals are respected.
How can bias in AI be addressed?
Addressing bias in AI involves multiple steps, including diverse and inclusive data collection, transparency in algorithms, regular auditing for biases, and involving diverse teams in the AI development process to ensure different perspectives are considered.
What are the principles of Ethical AI?
Common principles include transparency (understanding how AI systems make decisions), justice and fairness (avoiding bias and ensuring equality), non-maleficence (preventing harm), responsibility (accountability for AI’s impact), and privacy (protecting personal information).
How are governments and organizations promoting Ethical AI?
Governments and organizations are promoting Ethical AI through the development of guidelines, frameworks, and regulations that dictate the responsible use of AI. Initiatives like the EU’s Ethics Guidelines for Trustworthy AI and the OECD Principles on AI are examples of such efforts.
Can Ethical AI be achieved in practice?
Achieving Ethical AI is challenging but possible through collaborative efforts between developers, users, policymakers, and ethicists. It requires ongoing dialogue, education, and commitment to ethical standards, as well as the implementation of effective policies and oversight mechanisms.
The ultimate playground for sonic architects: Spunky combines streetwise aesthetics with cutting-edge audio manipulation tools.
Great breakdown! It’s clear how games like Super Ace blend luck and strategy, especially with wilds and free spins boosting the thrill and winning potential.
I enjoyed reading this article. Thanks for sharing your insights.
Great read! It’s fascinating how poker psychology mirrors creative tools like Ghibli Style with GPT-4o-both require patience, strategy, and an eye for detail to bring out the best results.
Online gaming thrives on security and smart play. Platforms like Jili No1 are setting a benchmark with AI-driven insights and a secure, user-friendly setup-ideal for both casual and serious players.
Great insights! Tools like Manus AI are reshaping automation, especially in data-driven fields. For a seamless workflow, check out DeerFlow AI.
Great read! It’s always fascinating to see how strategy and logic come into play in games like blackjack. For those looking to streamline their workflow and discover top AI tools, I highly recommend checking out the AI SEO Assistant – it’s a game-changer for optimizing your digital presence.
The MCP AI platform is a game-changer for developers, especially with how it enhances AI integration and decision-making. Exploring MCP Entertainment And Media shows its growing influence in creative industries. Exciting times ahead!
It’s amazing how game mods like Sprunki breathe new life into classics-especially with fresh beats and visuals that keep the creative fun going.
Ducking trains and collecting coins in Subway Surfers is pure adrenaline! It’s amazing how addictive the game can get. If you’re looking to jump in, check out Subway Surfers Games for more details.
Thanks for the insights! As a new player, I found Jiliph‘s AI-driven gameplay analysis really helpful in improving my strategy. The registration was smooth, and the game variety keeps things exciting!
E-sports and casino gaming both thrive on strategy and timing. Platforms like JLJL PH offer immersive experiences with live dealers and slots-perfect for fans looking to diversify their gaming portfolio.
If you’re into live dealers and top slots, check out SuperPH-they’ve got a smooth experience with great bonuses and reliable service. Worth a spin!
Great breakdown! It’s clear how crucial adaptability and emotional control are at the table. For a seamless poker experience, check out phwin99-it’s where strategy meets play.
Great post on roulette strategies-it’s all about probability and patience. For those who love deep analysis, the phdream vip insights could sharpen your edge in any competitive betting scenario.
Trying out JiliOK 777 was a hit-smooth sign-up, fun games, and that AI feel makes it exciting. A fresh twist on scratch cards!
Solid analysis! User experience is everything in modern betting. Platforms like PhDream11 login really highlight that – streamlined access is key. Good piece on anticipating player needs too! 👍
Understanding game mechanics is HUGE for consistent wins. It’s not just luck! Building a solid foundation, like with SuperPH26 Login, can really shift your perspective & improve results. Great article!
Thanks for the insights! I tried SuperPH and loved the live dealer games and smooth login process. Great resource for new players looking for a secure and fun platform!
Great insights! It’s fascinating how platforms like ph978 blend security, variety, and thrill into one seamless experience-perfect for both casual and serious players.
That’s a great point about deliberate practice! Building skills takes focus, and a solid platform helps. I’ve been checking out Pinas777 Online – the structured approach to mastery seems really promising, especially the emphasis on building a strong foundation. It’s more than just luck!
Interesting analysis! Seeing more platforms like PH987.Com Login emerge in the Asian market-strategic tools for players are key. Understanding game mechanics does seem to be the next level of engagement! Good read.
It’s easy to get carried away with online casinos, so responsible budgeting is key! Seeing platforms like phabc8 emphasize simple registration & verification is a good sign for player security, though still proceed with caution.
Transforming photos into Ghibli magic feels like a dream! I tried 지브리 AI and was amazed how easy it was to create that whimsical, storybook vibe-perfect for adding a touch of fantasy to my projects.
Mobile gaming is so different now! It’s all about that seamless experience, right? Really digging how platforms like playtime ph login are focusing on intuitive design – makes a huge difference when you’re on the go! Definitely a step up.
Interesting points! Navigating new platforms can be tricky, especially with verification – seen that with 789win surf. Security is key, but ease of use matters too, right? A good balance is crucial for wider adoption.
Smart bankroll management is key in any online game, and understanding the fundamentals is crucial. Resources like phlogin offer a structured approach to learning – beyond just playing, it’s about building skills! Definitely worth checking out for a solid foundation.
Smart bankroll management is key, especially with so many tempting options these days! Platforms like boss jili really emphasize variety – a little overwhelming, but fun! Finding a balance is crucial for long-term enjoyment. 🤔
Luck and strategy go hand in hand in online gaming, and platforms like Swerte99 get that balance right. Their live dealer games and slots offer real thrill without sacrificing user experience. Great read!
Scratch cards are such a fun, quick thrill! Reminds me of exploring new games – just signed up for JL Boss App and the variety is amazing! Check out boss jili – so many options, from classic slots to live dealer games. Definitely worth a look if you enjoy a bit of playful competition! ✨
Interesting analysis! Mobile gaming is definitely evolving fast. Seamless access is key – sounds like the jlboss app is aiming for that convenience. Quick logins & easy funding are huge wins for players on the go! 👍
Scratch cards are such a fun, quick thrill! It’s cool to see platforms like jboss offering so many gaming options – easy registration & multiple payment methods sound perfect for a casual player like me! 👍
Tui mới vào Bet666 login Password test thử thì quay cái là trúng, mà lúc đó mạng lag muốn xỉu 😂
Interesting read! Understanding player psychology is key, especially in a market like the Philippines where community matters. I noticed philwin games seem to prioritize that local connection – a smart move for building trust and engagement!
Pattern recognition in baccarat is fascinating – it’s about spotting subtle shifts, not guaranteed wins. JL Boss Tech’s AI game discovery at jk bose could actually help analyze your style – interesting approach to player data! Definitely a platform to watch.
Interesting analysis! The shift towards AI & blockchain in gaming is huge. Seeing platforms like 99wim game prioritize speed & security with local payment options is a smart move for the Vietnamese market – truly innovative!
Solid article! Understanding basic strategy is key, but a smooth platform matters too. Heard good things about JiliBoss – quick registration & great graphics. Check out jiliboss slot download for a premium experience – might help level up your game! 😉
That’s a fascinating take on longshot strategies! Seeing platforms like jl boss login prioritize secure account setup really builds trust – crucial for enjoying any online game responsibly. Interesting analysis!
Scratch cards are such a fun, quick thrill! It’s cool seeing platforms like jljlboss game offer so many options beyond just those – slots & live dealers too! Seems legit with that KYC process. 👍