Introduction
Embarking on a journey through the Machine Learning History Timeline offers a fascinating glimpse into the evolution of one of the most revolutionary technologies of our era that is an anchor of artificial intelligence. This blog post aims to unravel the rich information on machine learning’s past, tracing its roots from the initial concepts of artificial intelligence to the sophisticated, data-driven algorithms that define the field today.
As we navigate through the milestones that have marked the progress of machine learning, we gain not only a deeper appreciation for the ingenuity and perseverance of researchers and developers but also an insightful perspective on how this technology has become intertwined with the fabric of modern society. We will explore the pivotal moments and breakthroughs that have shaped machine learning, illuminating the path from its inception to the cutting-edge advancements that continue to push the boundaries of what machines can learn and achieve.
Table of Contents
Key Milestones on Machine Learning Timeline
Year | Milestone |
---|---|
1950s | Early Concepts: Alan Turing proposes the Turing Test as a criterion of intelligence, sparking discussions on “thinking machines”. |
1952 | First Neural Network for Computers: Arthur Samuel develops a self-learning program, the precursor to machine learning algorithms, for playing checkers. |
1957 | The Perceptron: Frank Rosenblatt invents the Perceptron, an early neural network, introducing the concept of trainable algorithms. |
1967 | Nearest Neighbor Algorithm: The nearest neighbor algorithm was written, allowing patterns to be classified based on the closest stored patterns. |
1980s | Backpropagation: The development of the backpropagation algorithm enables practical training of multi-layer neural networks, significantly advancing the neural network field. |
1990s | Support Vector Machines: The introduction of Support Vector Machines (SVM) offers a new powerful tool for data classification. |
1997 | Deep Blue Beats Kasparov: IBM’s Deep Blue becomes the first computer to defeat a world chess champion, Garry Kasparov, showcasing the potential of AI. |
2006 | Deep Learning Era Begins: Geoffrey Hinton and colleagues introduce a fast learning algorithm for deep belief nets, reigniting interest in deep neural networks. |
2012 | AlexNet: Alex Krizhevsky’s AlexNet wins the ImageNet challenge by a large margin, demonstrating the power of deep learning in image recognition. |
2014 | Generative Adversarial Networks (GANs): Ian Goodfellow and his colleagues introduce GANs, further expanding the capabilities of generative models. |
2016 | AlphaGo: Google DeepMind’s AlphaGo defeats Lee Sedol, a world champion Go player, marking a significant achievement in AI for complex game solving. |
2020s | GPT-3 and Beyond: OpenAI introduces GPT-3, showcasing advanced natural language processing capabilities and fueling further exploration in generative AI models. |
Here are detailed explanations for each milestone in the “Machine Learning History Timeline”:
1950s: Early Concepts
Alan Turing proposes the Turing Test in his paper “Computing Machinery and Intelligence,” questioning whether machines can think. The test evaluates a machine’s ability to exhibit intelligent behavior indistinguishable from that of a human.
1952: First Neural Network for Computers
Arthur Samuel develops a self-learning program to play checkers, demonstrating that machines can learn from experience. His work lays foundational concepts for future machine learning algorithms.
1957: The Perceptron
Frank Rosenblatt invents the Perceptron, an early form of neural network, at the Cornell Aeronautical Laboratory. The Perceptron was designed to recognize basic patterns and shapes, introducing trainable algorithms.
1967: Nearest Neighbor Algorithm
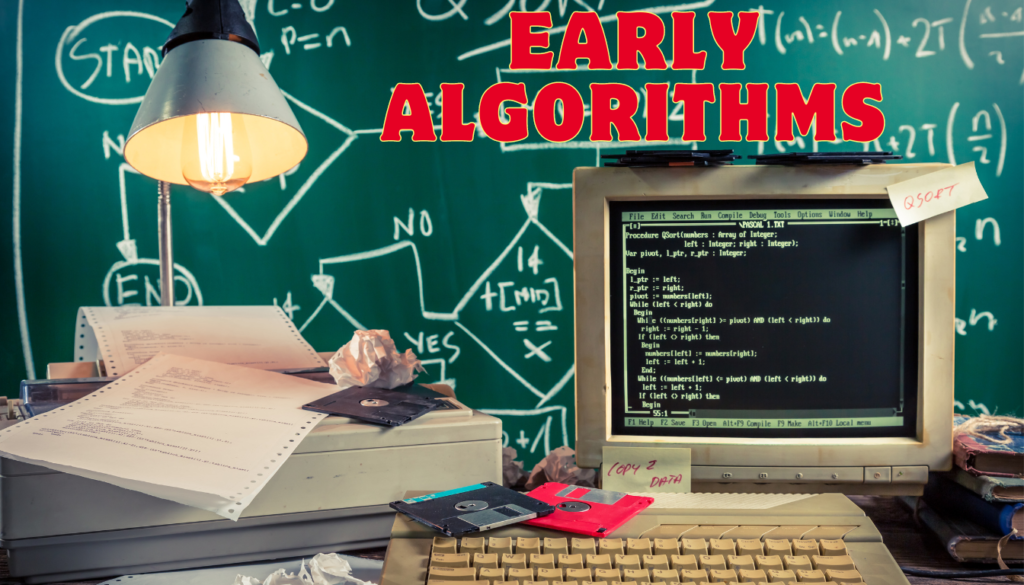
The nearest neighbor algorithm was formalized, allowing classification of patterns based on the most similar stored examples. This simple yet effective approach became a staple in pattern recognition.
1980s: Backpropagation
The development of the backpropagation algorithm by multiple researchers independently, including Rumelhart, Hinton, and Williams, enables the practical training of multi-layer neural networks, significantly advancing the capabilities of neural networks.
1990s: Support Vector Machines
Vladimir Vapnik and colleagues introduce Support Vector Machines (SVM), providing a robust and efficient methodology for data classification and regression analysis.
1997: Deep Blue Beats Kasparov
IBM’s Deep Blue becomes the first computer system to defeat a reigning world chess champion, Garry Kasparov. This event highlights the potential of AI systems to perform at and beyond human expert levels in specific tasks.
2006: Deep Learning Era Begins
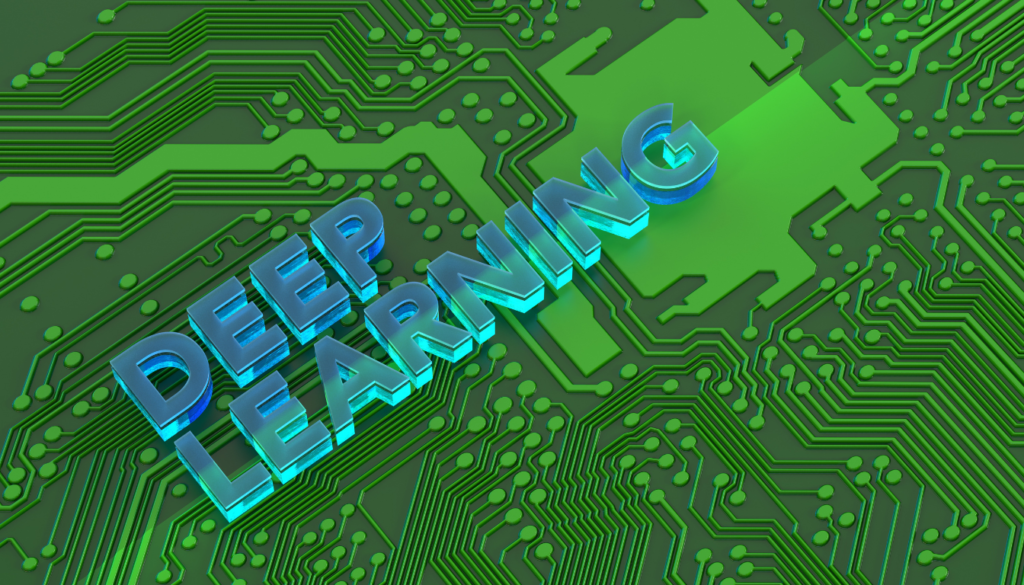
Geoffrey Hinton, Ruslan Salakhutdinov, and colleagues introduce a fast learning algorithm for deep belief networks, marking the beginning of the modern deep learning era. This work reinvigorates interest in neural networks.
2012: AlexNet
Developed by Alex Krizhevsky, Ilya Sutskever, and Geoffrey Hinton, AlexNet significantly outperforms other submissions in the ImageNet Large Scale Visual Recognition Challenge (ILSVRC), demonstrating the effectiveness of deep neural networks in image classification.
2014: Generative Adversarial Networks (GANs)
Ian Goodfellow and his colleagues introduce Generative Adversarial Networks (GANs), a novel framework for training generative models, leading to significant advancements in creating realistic images, videos, and voice audio.
2016: AlphaGo
Google DeepMind’s AlphaGo defeats Lee Sedol in the game of Go, a feat previously considered a decade away. AlphaGo’s success demonstrates the power of combining deep neural networks and reinforcement learning to solve complex problems.
2020s: GPT-3 and Beyond
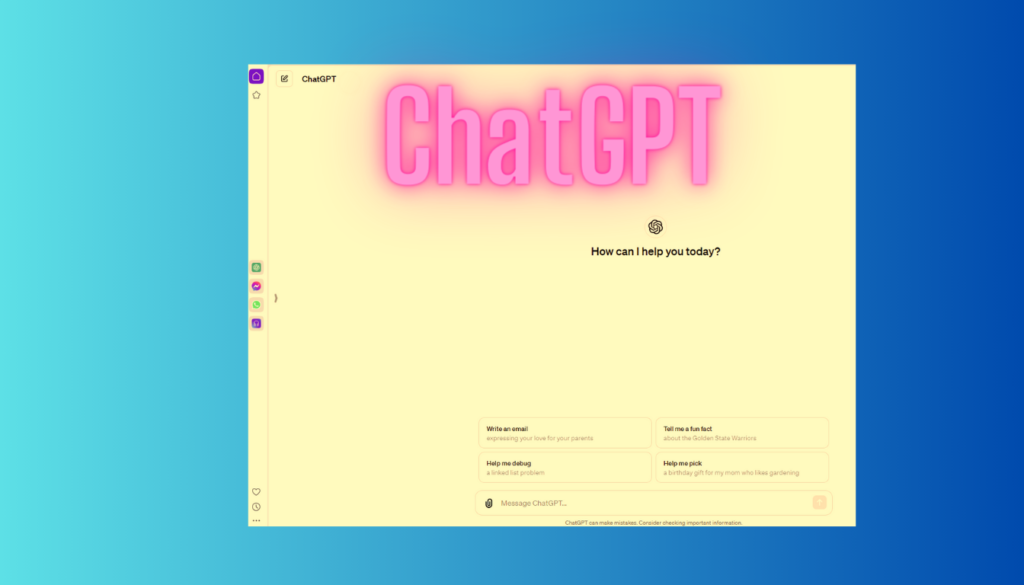
OpenAI introduces GPT-3, the third generation of the Generative Pre-trained Transformer, showcasing remarkable natural language processing capabilities, from writing essays to coding, and ignites further research and development in generative AI models.
Conclusion and Final Thoughts
The journey through the Machine Learning History Timeline showcases an impressive saga of human ingenuity, relentless curiosity, and technological advancements. From the early theoretical foundations laid down in the 1950s to the cutting-edge developments of today, machine learning has grown from a speculative vision to a transformative force across industries and aspects of daily life.
Reflecting on the Past: The milestones we’ve traversed reveal not just the evolution of algorithms and models but also a deepening understanding of what machines are capable of achieving. Each breakthrough, from the Perceptron to GPT-3, stands as a testament to the collaborative spirit of the scientific community and its drive to push the boundaries of possibility.
Embracing the Present: Today, machine learning is not a distant marvel but a ubiquitous tool. Its applications, from enhancing medical diagnostics to driving autonomous vehicles, from crafting personalized digital experiences to solving complex global challenges, underscore its integral role in shaping the present and future.
Anticipating the Future: As we look forward, the trajectory of machine learning is poised for even greater leaps. With the advent of quantum computing, AI ethics, and continual breakthroughs in algorithms and computing power, the next chapters of this timeline promise to be even more groundbreaking. The journey of machine learning is far from complete, and its full potential is yet to be realized.
The Role of Each One of Us: Engaging with the history of machine learning invites us to participate in this ongoing journey. Whether as developers, researchers, enthusiasts, or simply informed citizens, we each have a role in shaping how this technology evolves and is applied. By understanding its history, we are better equipped to contribute to a future where machine learning continues to serve as a force for good, enhancing human capabilities and addressing the challenges of our times.
In closing, the Machine Learning History Timeline is not just a record of past achievements; it’s a beacon for future explorations and innovations. As we stand on the shoulders of giants, we are inspired to dream bigger, push further, and continue the pursuit of knowledge that drives the remarkable field of machine learning forward.
Fascinating insights! The way you articulate ideas reflects what Sprunki OC brings to artists. Speaking of creativity, Sprunki OC offers unprecedented creative freedom. The Sprunki OC platform is revolutionary.
What you’ve written here feels like a conversation with a wise friend, offering insights that are both gentle and profound.
Slot games like Super Ace Jili offer a perfect mix of luck and strategy. The 1024 ways to win and wild substitutions make each spin thrilling-definitely one to try for new and seasoned players alike.
Online gaming platforms like Jili offer great variety and convenience, especially with AI features boosting gameplay on sites like Jili777 Login. It’s impressive how tech is reshaping betting experiences.
This article brilliantly captures the nuances of data-driven betting strategies. It’s fascinating how tools like Manus AI are revolutionizing automation in such detail-oriented fields.
Great insights on leveraging data for better betting outcomes. For those looking to dive deeper into AI-driven analytics, check out the AI Analytics Assistant-a powerful tool for smart decision-making.